Tuesday, 6 May 2025
Putting data governance at the heart of a customer redress scheme
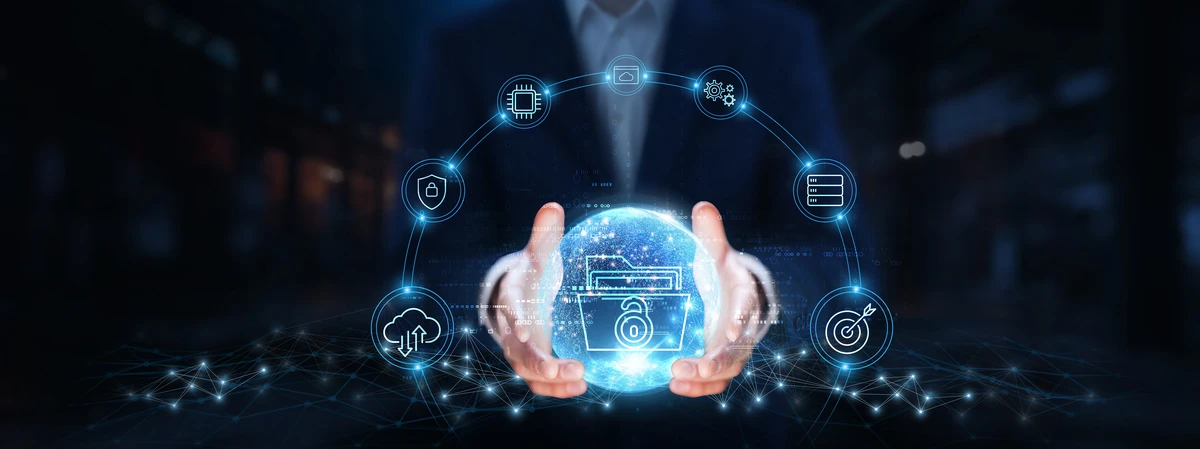
Soon, motor finance providers will learn of the Supreme Court’s ruling on the lawfulness of hidden commission in car finance agreements.
If the Court finds that lenders’ failure to fully inform customers about their car finance commission was unlawful, finance industry regulator the Financial Conduct Authority (FCA) is likely to consult on a redress scheme. This would require the finance sector to compensate hundreds of thousands of consumers.
The FCA is already considering a redress scheme in response to complaints about car finance discretionary commission arrangements (DCAs), also relating to hidden commission. A redress scheme on this scale would have significant financial implications for the finance sector; it would also put providers’ data teams and systems under severe pressure. The FCA’s review last year of DCAs – which saw it assessing thousands of records spanning 14 years – hints at some of the challenges for lenders.
The FCA reported that, while firms engaged with them constructively, many struggled to supply the data needed within its requested timescale. Reasons for delays in providing data, it said, included, “firms not keeping older data, and data being stored on multiple systems, or being spread between lenders and brokers”.
With this in mind, we are returning to data for the last in our series of blogs for lenders planning for potential remediation projects. Drawing on Talan Data’s experience in best practice remediation projects, this blog sets out why data governance matters.
A key decision for lenders is the level of support needed in developing a remediation project and the extent to which that should be delivered by specialist partners. Potential risks, such as non-compliance, regulatory fines, data security breaches and inaccurate decisions and payouts, can be successfully mitigated by working with expert specialists.
Talan Data and AI’s work is informed by two decades of experience in managing and supporting large-scale remediation programmes, including complex redress and data quality initiatives for the financial services sector. We have a 200-strong team of data specialists ready to prepare and support responses to an anticipated redress scheme – taking lenders from strategy through to implementation.
In this blog series, we have outlined the essential characteristics of a redress scheme, including the importance of data accuracy and the effective deployment of artificial intelligence. You can read more here: